Metrics
The cortex get
and cortex get API_NAME
commands display the request time (averaged over the past 2 weeks) and response code counts (summed over the past 2 weeks) for your APIs:
The cortex get API_NAME
command also provides a link to a Grafana dashboard:
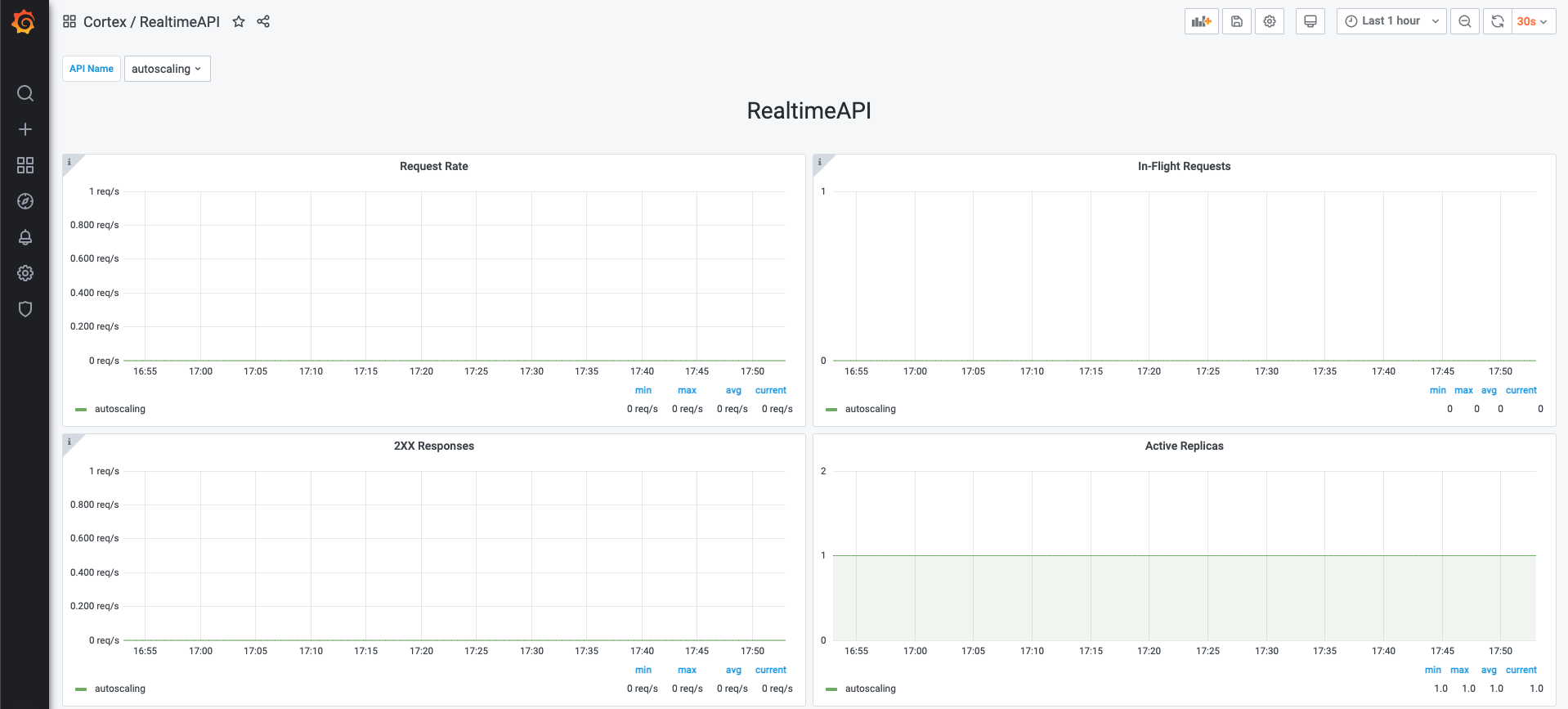
Metrics in the dashboard
Panel | Description | Note |
Request Rate | Request rate, computed over every minute, of an API | |
In Flight Request | Active in-flight requests for an API. | In-flight requests are recorded every 10 seconds, which will correspond to the minimum resolution. |
Active Replicas | Active replicas for an API | |
2XX Responses | Request rate, computed over a minute, for responses with status code 2XX of an API | |
4XX Responses | Request rate, computed over a minute, for responses with status code 4XX of an API | |
5XX Responses | Request rate, computed over a minute, for responses with status code 5XX of an API | |
p99 Latency | 99th percentile latency, computed over a minute, for an API | Value might not be accurate because the histogram buckets are not dynamically set. |
p90 Latency | 90th percentile latency, computed over a minute, for an API | Value might not be accurate because the histogram buckets are not dynamically set. |
p50 Latency | 50th percentile latency, computed over a minute, for an API | Value might not be accurate because the histogram buckets are not dynamically set. |
Average Latency | Average latency, computed over a minute, for an API |
Custom user metrics
It is possible to export custom user metrics by adding the metrics_client
argument to the predictor constructor. Below there is an example of how to use the metrics client with the PythonPredictor
type. The implementation would be similar to other predictor types.
Refer to the observability documentation for more information on custom metrics.
Note: The metrics client uses the UDP protocol to push metrics, to be fault tolerant, so if it fails during a metrics push there is no exception thrown.
Last updated